ローカルで動いたTensorflow環境をTPUに持って行くとすんなり動かない。動いた物を集めながら、差分を少しずつでも調べていかないと前に進まない感じがする。少しずつ環境をつくっています。やりたいことがいっぱいあるがもどかしい。
TPUを使う
Kerasを使わずにTPUを使ったサンプル。これはあっさりと動きました。
import os
import tensorflow as tf
from tensorflow.contrib import tpu
from tensorflow.examples.tutorials.mnist import input_data
MAX_EPOCH = 50
mnist = input_data.read_data_sets("MNIST_data/", one_hot=True)
num_input = 28 * 28 * 1
num_classes = 10
x_ = tf.placeholder("float", shape=[None, num_input], name='X')
y_ = tf.placeholder("float", shape=[None, num_classes], name='Y')
is_training = tf.placeholder(tf.bool)
x_image = tf.reshape(x_, [-1, 28, 28, 1])
conv1 = tf.layers.conv2d(inputs=x_image, filters=32, kernel_size=(5, 5), padding='same', activation=tf.nn.relu)
pool1 = tf.layers.max_pooling2d(inputs=conv1, pool_size=(2, 2), strides=2)
conv2 = tf.layers.conv2d(inputs=pool1, filters=64, kernel_size=(5, 5), padding='same', activation=tf.nn.relu)
pool2 = tf.layers.max_pooling2d(inputs=conv2, pool_size=(2, 2), strides=2)
pool2_flat = tf.reshape(pool2, shape=[-1, 7 * 7 * 64])
dense = tf.layers.dense(inputs=pool2_flat, units=1024, activation=tf.nn.relu)
dropout = tf.layers.dropout(inputs=dense, rate=0.4, training=is_training)
logits = tf.layers.dense(inputs=dropout, units=10)
cross_entropy = tf.reduce_mean(tf.nn.softmax_cross_entropy_with_logits_v2(logits=logits, labels=y_))
train_step = tf.train.AdamOptimizer(1e-4).minimize(cross_entropy)
correct_prediction = tf.equal(tf.argmax(logits, 1), tf.argmax(y_, 1))
accuracy = tf.reduce_mean(tf.cast(correct_prediction, tf.float32))
tpu_grpc_url = tf.contrib.cluster_resolver.TPUClusterResolver(tpu=[os.environ['TPU_NAME']])
strategy = tf.contrib.tpu.TPUDistributionStrategy(tpu_grpc_url)
init = tf.global_variables_initializer()
tf.summary.scalar('softmax_cross_entropy', cross_entropy)
tf.summary.scalar('accuracy', accuracy)
merged = tf.summary.merge_all()
with tf.Session() as sess:
with tf.name_scope('summary'):
writer = tf.summary.FileWriter('./logs_tpu', sess.graph)
sess.run(init)
for i in range(MAX_EPOCH):
batch = mnist.train.next_batch(50)
train_accuracy = accuracy.eval(session=sess, feed_dict={x_: batch[0], y_: batch[1], is_training: True})
print("step = %d, training accuracy = %g" % (i, train_accuracy))
s = sess.run(merged, feed_dict={x_: batch[0], y_: batch[1], is_training: False})
writer.add_summary(s, i)
train_step.run(session=sess, feed_dict={x_: batch[0], y_: batch[1], is_training: False})
print("Test Accuracy:", sess.run(accuracy, feed_dict={x_: mnist.test.images, y_: mnist.test.labels, is_training: False}))
tpu.shutdown_system()
Tensorboardを使う
ここを参考に6006のfirewall ruleを追加することで、GCPでの学習結果をtensorboardで確認できます。
https://qiita.com/tk_01/items/307716a680460f8dbe17
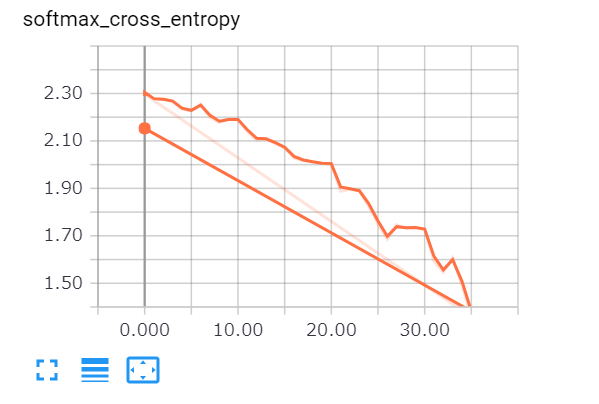
どうやら上手く学習できているようです。